CalculateChiSquared v1¶
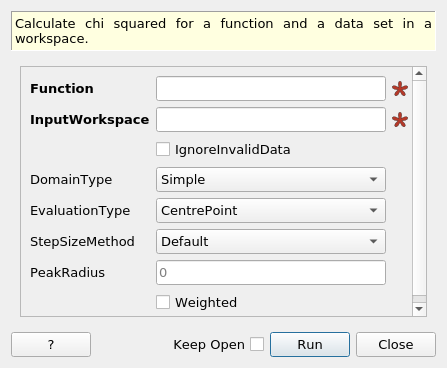
CalculateChiSquared dialog.¶
Summary¶
Calculate chi squared for a function and a data set in a workspace.
See Also¶
Properties¶
Name |
Direction |
Type |
Default |
Description |
---|---|---|---|---|
Function |
InOut |
Function |
Mandatory |
Parameters defining the fitting function and its initial values |
InputWorkspace |
Input |
Mandatory |
Name of the input Workspace |
|
IgnoreInvalidData |
Input |
boolean |
False |
Flag to ignore infinities, NaNs and data with zero errors. |
DomainType |
Input |
string |
Simple |
The type of function domain to use: Simple, Sequential, or Parallel. Allowed values: [‘Simple’, ‘Sequential’, ‘Parallel’] |
EvaluationType |
Input |
string |
CentrePoint |
The way the function is evaluated on histogram data sets. If value is “CentrePoint” then function is evaluated at centre of each bin. If it is “Histogram” then function is integrated within the bin and the integrals returned. Allowed values: [‘CentrePoint’, ‘Histogram’] |
StepSizeMethod |
Input |
string |
Default |
The way the step size is calculated for numerical derivatives. See the section about step sizes in the Fit algorithm documentation to understand the difference between “Default” and “Sqrt epsilon”. Allowed values: [‘Default’, ‘Sqrt epsilon’] |
PeakRadius |
Input |
number |
0 |
A value of the peak radius the peak functions should use. A peak radius defines an interval on the x axis around the centre of the peak where its values are calculated. Values outside the interval are not calculated and assumed zeros.Numerically the radius is a whole number of peak widths (FWHM) that fit into the interval on each side from the centre. The default value of 0 means the whole x axis. |
ChiSquared |
Output |
number |
Output value of chi squared. |
|
ChiSquaredDividedByDOF |
Output |
number |
Output value of chi squared divided by the number of degrees of freedom (NofData - nOfParams). |
|
ChiSquaredDividedByNData |
Output |
number |
Output value of chi squared divided by the number of data points). |
|
ChiSquaredWeighted |
Output |
number |
Output value of weighted chi squared. |
|
ChiSquaredWeightedDividedByDOF |
Output |
number |
Output value of weighted chi squared divided by the number of degrees of freedom (NofData - nOfParams). |
|
ChiSquaredWeightedDividedByNData |
Output |
number |
Output value of weighted chi squared divided by the number of data points). |
|
Weighted |
Input |
boolean |
False |
Option to use the weighted chi squared in error estimation. Default is false. |
Description¶
Calculates a measure of the goodness of a fit in four ways.
The ChiSquared property returns the sum of squares of differences between the calculated and measured values:
where
The ChiSquaredDividedByDOF is ChiSquared divided by the number of degrees of freedom (DOF):
The ChiSquaredWeighted property sums the squares of the differences divided by the data errors:
Finally, ChiSquaredWeightedDividedByDOF is
Usage¶
Example 1
import numpy as np
# Create a data set
x = np.linspace(0,1,10)
y = 1.0 + 2.0 * x
e = np.sqrt(y)
ws = CreateWorkspace(DataX=x, DataY=y, DataE=e)
# Define a function
func = 'name=LinearBackground,A0=1.1,A1=1.9'
# Calculate the chi squared
chi2,chi2dof,chi2ndata,chi2W,chi2Wdof,chi2Wndata = CalculateChiSquared(func,ws)
print('Chi squared is {:.13f}'.format(chi2))
print('Chi squared / DOF is {:.14f}'.format(chi2dof))
print('Chi squared / NDATA is {:.14f}'.format(chi2ndata))
print('Chi squared weighted is {:.11f}'.format(chi2W))
print('Chi squared weighted / DOF is {:.14f}'.format(chi2Wdof))
print('Chi squared weighted / NDATA is {:.12f}'.format(chi2Wndata))
# Define a function that models the data exactly
func = 'name=LinearBackground,A0=1.0,A1=2.0'
# Calculate the chi squared
chi2,chi2dof,chi2ndata,chi2W,chi2Wdof,chi2Wndata = CalculateChiSquared(func,ws)
print('Chi squared is {:.1f}'.format(chi2))
print('Chi squared / DOF is {:.1f}'.format(chi2dof))
print('Chi squared / NDATA is {:.1f}'.format(chi2ndata))
print('Chi squared weighted is {:.1f}'.format(chi2W))
print('Chi squared weighted / DOF is {:.1f}'.format(chi2Wdof))
print('Chi squared weighted / NDATA is {:.1f}'.format(chi2Wndata))
Output:
Chi squared is 0.0351851851852
Chi squared / DOF is 0.00439814814815
Chi squared / NDATA is 0.00351851851852
Chi squared weighted is 0.02660287840
Chi squared weighted / DOF is 0.00332535979971
Chi squared weighted / NDATA is 0.002660287840
Chi squared is 0.0
Chi squared / DOF is 0.0
Chi squared / NDATA is 0.0
Chi squared weighted is 0.0
Chi squared weighted / DOF is 0.0
Chi squared weighted / NDATA is 0.0
Categories: AlgorithmIndex | Optimization
Source¶
C++ header: CalculateChiSquared.h
C++ source: CalculateChiSquared.cpp