FindPeaksAutomatic v1¶
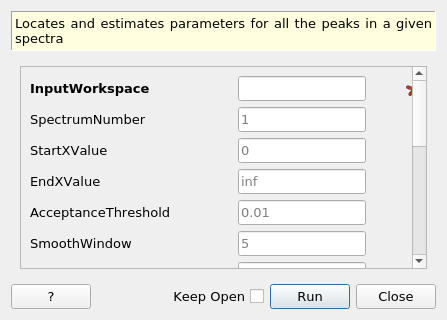
FindPeaksAutomatic dialog.¶
Summary¶
Locates and estimates parameters for all the peaks in a given spectra
See Also¶
FitGaussianPeaks, FindPeaks, FindPeaksMD, FindSXPeaks, FitPeak, FitPeaks
Properties¶
Name |
Direction |
Type |
Default |
Description |
---|---|---|---|---|
InputWorkspace |
Input |
Mandatory |
Workspace with peaks to be identified |
|
SpectrumNumber |
Input |
number |
1 |
Spectrum number to use |
StartXValue |
Input |
number |
0 |
Value of X to start the search from |
EndXValue |
Input |
number |
Optional |
Value of X to stop the search to |
AcceptanceThreshold |
Input |
number |
0.01 |
Threshold for considering a peak significant, the exact meaning of the value depends on the cost function used and the data to be fitted. Good values might be about 1-10 for poisson cost and 0.0001-0.01 for chi2 |
SmoothWindow |
Input |
number |
5 |
Half size of the window used to find the background values to subtract |
BadPeaksToConsider |
Input |
number |
20 |
Number of peaks that do not exceed the acceptance threshold to be searched before terminating. This is useful because sometimes good peaks can be found after some bad ones. However setting this value too high will make the search much slower. |
UsePoissonCost |
Input |
boolean |
False |
Use a probabilistic approach to find the cost of a fit instead of using chi2. |
FitToBaseline |
Input |
boolean |
False |
Use a probabilistic approach to find the cost of a fit instead of using chi2. |
EstimatePeakSigma |
Input |
number |
3 |
A rough estimate of the standard deviation of the gaussian used to fit the peaks |
MinPeakSigma |
Input |
number |
0.5 |
Minimum value for the standard deviation of a peak |
MaxPeakSigma |
Input |
number |
30 |
Maximum value for the standard deviation of a peak |
PlotPeaks |
Input |
boolean |
False |
Plot the position of the peaks found by the algorithm |
PlotBaseline |
Input |
boolean |
False |
Plot the baseline as calculated by the algorithm |
PeakPropertiesTableName |
Output |
peak_table |
Name of the table containing the properties of the peaks |
|
RefitPeakPropertiesTableName |
Output |
refit_peak_table |
Name of the table containing the properties of the peaks that had to be fitted twice as the first time the error was unreasonably large |
|
OutputWorkspace |
Output |
workspace_with_errors |
Workspace containing the same data as the input one, with errors added if not present from the beginning |
Description¶
The algorithm takes a workspace containing a spectrum and will attempt to find the number and parameters of all the peaks (assumed to be gaussian).
To do so the algorithm performs the following:
The data is loaded, invalid values discarded and the run is cropped to the user defined window.
If no error value is provided the error is calculated as the square root of the y values.
The morphological operations of erosion and dilation are used to calculate a baseline. If the option FitToBaseline is selected, the following steps will be performed on the baseline. Otherwise the baseline is subtracted from the data.
All peaks (including noise) are identified and sorted by relative height with respect to the background.
The cost function is evaluated against a flat background (i.e. no peak hypothesis).
The best peak found in step #4 and not yet considered is added to the list of peaks to be fitted. A fit is performed using the Fit algorithm and the cost function is evaluated on the result.
If the newly calculated cost is compared to the previous best one. This is done differently for poisson and
costs: : if the new cost is lower than the previous best and the relative difference is reater than AcceptanceThreshold the peak is considered valid.poisson: if the difference between the new cost and the previous best is greater than
the peak is considered valid
If more than BadPeaksToConsider invalid peaks have been encountered since the last valid peak then the program terminates and the list of valid peaks is returned. Otherwise the procedure is repeated from step #6.
To fit a list of peaks and calculate the cost algorithm FitGaussianPeaks is used. If the option PlotPeaks is selected then after completing the algorithm a plot of the input data is generated and the peaks marked with red x. If the option PlotBaseline is also selected then the baseline will also be shown. This is particularly useful when tuning the value of SmoothWindow (see below).
Improving the fit¶
The result of the fit is strongly dependent on the parameter chosen. Unfortunately these depend on the particular data to be fitted. Therefore, while the default choice will often generate reasonable answers it is often necessary to adjust some of them.
The most important to this effect are:
AcceptanceThreshold, the value of this depends on the cost function used. For
, reasonable values range between 0.0001-0.1. For poisson cost, reasonable value range between 1-100. In both cases, higher values correspond to stronger constraints, and will lead to less peaks found. Conversely, lower values of the parameter will include more peaks, this causes the algorithm to slow down and to include more undesired peaks in the final output.SmoothWindow, this affects the calculation of the baseline. Setting the parameter to 0 leaves the data unchanged. When subtracting a baseline found with low values of the parameter, all but the sharpest peaks will be removed, eliminating noise most effectively but also potentially getting rid of peaks of interest. Conversely, higher values will be less aggressive, leaving broader peaks and noise features to be fitted.
BadPeaksToConsider, this is the number of invalid peaks that can be encountered since the last valid one before the algorithm is terminated. If the parameter is too low, there is a possibility that valid peaks will be discarded because some noise features were previously encountered. However, higher values will strongly affect the performance and will tend to introduce unwanted noise in the final peak count.
EstimatePeakSigma, this is an initial guess for the standard deviation of the peaks in the data. It becomes especially important when trying to differentiate closely spaced features, where lower values of this parameter will usually perform better than higher ones.
Example - Find two gaussian peaks on an exponential background with added noise
# Function for a gaussian peak
def gaussian(xvals, centre, height, sigma):
exp_val = (xvals - centre) / (np.sqrt(2) * sigma)
return height * np.exp(-exp_val * exp_val)
np.random.seed(1234)
# Creating two peaks on an exponential background with gaussian noise
x_values = np.linspace(0, 1000, 1001)
centre = [250, 750]
height = [350, 200]
width = [3, 2]
y_values = gaussian(x_values, centre[0], height[0], width[0])
y_values += gaussian(x_values, centre[1], height[1], width[1])
y_values_low_noise = y_values + np.abs(400 * np.exp(-0.005 * x_values)) + 30 + 0.1*np.random.randn(len(x_values))
y_values_high_noise = y_values + np.abs(400 * np.exp(-0.005 * x_values)) + 30 + 10*np.random.randn(len(x_values))
low_noise_ws = CreateWorkspace(DataX=x_values, DataY=y_values_low_noise, DataE=np.sqrt(y_values_low_noise))
high_noise_ws = CreateWorkspace(DataX=x_values, DataY=y_values_high_noise, DataE=np.sqrt(y_values_high_noise))
# Fitting the data with low noise
FindPeaksAutomatic(InputWorkspace=low_noise_ws,
AcceptanceThreshold=0.2,
SmoothWindow=30,
EstimatePeakSigma=2,
MaxPeakSigma=5,
PlotPeaks=False,
PeakPropertiesTableName='properties',
RefitPeakPropertiesTableName='refit_properties')
peak_properties = mtd['properties']
peak_low1 = peak_properties.row(0)
peak_low2 = peak_properties.row(1)
# Fitting the data with strong noise
FindPeaksAutomatic(InputWorkspace=high_noise_ws,
AcceptanceThreshold=0.1,
SmoothWindow=30,
EstimatePeakSigma=2,
MaxPeakSigma=5,
PlotPeaks=False,
PeakPropertiesTableName='properties',
RefitPeakPropertiesTableName='refit_properties')
peak_properties = mtd['properties']
peak_high1 = peak_properties.row(0)
peak_high2 = peak_properties.row(1)
print('Low noise')
print('Peak 1: centre={:.2f}+/-{:.2f}, height={:.2f}+/-{:.2f}, sigma={:.2f}+/-{:.2f}'
.format(peak_low1['centre'], peak_low1['error centre'],
peak_low1['height'], peak_low1['error height'],
peak_low1['sigma'], peak_low1['error sigma']))
print('Peak 2: centre={:.2f}+/-{:.2f}, height={:.2f}+/-{:.2f}, sigma={:.2f}+/-{:.2f}'
.format(peak_low2['centre'], peak_low2['error centre'],
peak_low2['height'], peak_low2['error height'],
peak_low2['sigma'], peak_low2['error sigma']))
print('')
print('Strong noise')
print('Peak 1: centre={:.2f}+/-{:.2f}, height={:.2f}+/-{:.2f}, sigma={:.2f}+/-{:.2f}'
.format(peak_high1['centre'], peak_high1['error centre'],
peak_high1['height'], peak_high1['error height'],
peak_high1['sigma'], peak_high1['error sigma']))
print('Peak 2: centre={:.2f}+/-{:.2f}, height={:.2f}+/-{:.2f}, sigma={:.2f}+/-{:.2f}'
.format(peak_high2['centre'], peak_high2['error centre'],
peak_high2['height'], peak_high2['error height'],
peak_high2['sigma'], peak_high2['error sigma']))
Output:
Low noise
Peak 1: centre=250.01+/-0.09, height=352.47+/-10.91, sigma=3.06+/-0.08
Peak 2: centre=750.00+/-0.09, height=200.03+/-9.13, sigma=2.01+/-0.07
Strong noise
Peak 1: centre=250.00+/-0.09, height=360.33+/-10.83, sigma=3.11+/-0.08
Peak 2: centre=749.90+/-0.08, height=194.86+/-7.82, sigma=2.47+/-0.07
Example - Find 2 different gaussian peaks on a flat background with added noise
# Function for a gaussian peak
def gaussian(xvals, centre, height, sigma):
exp_val = (xvals - centre) / (np.sqrt(2) * sigma)
return height * np.exp(-exp_val * exp_val)
np.random.seed(4321)
# Creating two peaks on a flat background with gaussian noise
x_values = np.array([np.linspace(0, 1000, 1001), np.linspace(0, 1000, 1001)], dtype=float)
centre = np.array([[250, 750], [100, 600]], dtype=float)
height = np.array([[350, 200], [400, 500]], dtype=float)
width = np.array([[10, 5], [3, 2]], dtype=float)
y_values = np.array([gaussian(x_values[0], centre[0, 0], height[0, 0], width[0, 0]),
gaussian(x_values[1], centre[1, 0], height[1, 0], width[1, 0])])
y_values += np.array([gaussian(x_values[0], centre[0, 1], height[0, 1], width[0, 1]),
gaussian(x_values[1], centre[1, 1], height[1, 1], width[1, 1])])
y_values_low_noise = y_values + np.abs(200 + 0.1*np.random.randn(*x_values.shape))
y_values_high_noise = y_values + np.abs(200 + 5*np.random.randn(*x_values.shape))
low_noise_ws = CreateWorkspace(DataX=x_values, DataY=y_values_low_noise, DataE=np.sqrt(y_values_low_noise),NSpec = 2)
high_noise_ws = CreateWorkspace(DataX=x_values, DataY=y_values_high_noise, DataE=np.sqrt(y_values_high_noise),NSpec =2)
# Fitting the data with low noise
FindPeaksAutomatic(InputWorkspace=low_noise_ws,
SpectrumNumber = 2,
AcceptanceThreshold=0.2,
SmoothWindow=30,
EstimatePeakSigma=2,
MaxPeakSigma=5,
PlotPeaks=False,
PeakPropertiesTableName='properties',
RefitPeakPropertiesTableName='refit_properties')
peak_properties = mtd['properties']
peak_low1 = peak_properties.row(0)
peak_low2 = peak_properties.row(1)
# Fitting the data with strong noise
FindPeaksAutomatic(InputWorkspace=high_noise_ws,
SpectrumNumber = 2,
AcceptanceThreshold=0.1,
SmoothWindow=30,
EstimatePeakSigma=2,
MaxPeakSigma=5,
PlotPeaks=False,
PeakPropertiesTableName='properties',
RefitPeakPropertiesTableName='refit_properties')
peak_properties = mtd['properties']
peak_high1 = peak_properties.row(0)
peak_high2 = peak_properties.row(1)
print('Low noise')
print('Peak 1: centre={:.2f}+/-{:.2f}, height={:.2f}+/-{:.2f}, sigma={:.2f}+/-{:.2f}'
.format(peak_low1['centre'], peak_low1['error centre'],
peak_low1['height'], peak_low1['error height'],
peak_low1['sigma'], peak_low1['error sigma']))
print('Peak 2: centre={:.2f}+/-{:.2f}, height={:.2f}+/-{:.2f}, sigma={:.2f}+/-{:.2f}'
.format(peak_low2['centre'], peak_low2['error centre'],
peak_low2['height'], peak_low2['error height'],
peak_low2['sigma'], peak_low2['error sigma']))
print('')
print('Strong noise')
print('Peak 1: centre={:.2f}+/-{:.2f}, height={:.2f}+/-{:.2f}, sigma={:.2f}+/-{:.2f}'
.format(peak_high1['centre'], peak_high1['error centre'],
peak_high1['height'], peak_high1['error height'],
peak_high1['sigma'], peak_high1['error sigma']))
print('Peak 2: centre={:.2f}+/-{:.2f}, height={:.2f}+/-{:.2f}, sigma={:.2f}+/-{:.2f}'
.format(peak_high2['centre'], peak_high2['error centre'],
peak_high2['height'], peak_high2['error height'],
peak_high2['sigma'], peak_high2['error sigma']))
Output:
Low noise
Peak 1: centre=100.00+/-0.09, height=400.19+/-12.18, sigma=3.00+/-0.08
Peak 2: centre=600.00+/-0.06, height=500.20+/-16.01, sigma=2.00+/-0.06
Strong noise
Peak 1: centre=100.00+/-0.10, height=377.97+/-0.02, sigma=3.35+/-0.07
Peak 2: centre=600.00+/-0.06, height=503.59+/-15.68, sigma=2.08+/-0.06
Categories: AlgorithmIndex | Optimization\PeakFinding
Source¶
Python: FindPeaksAutomatic.py